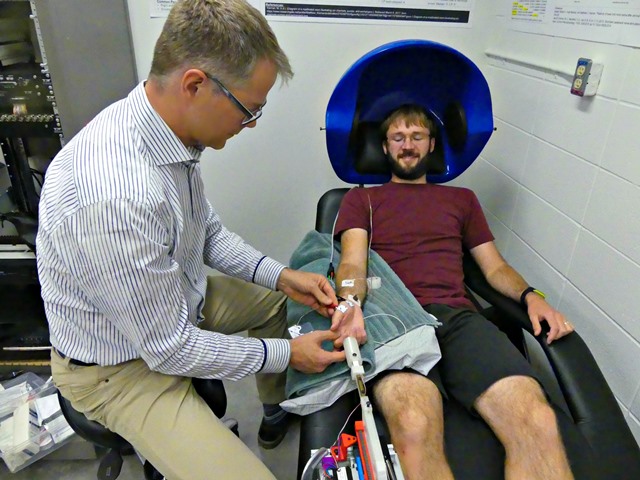
Dr. Jones simulates electrical diagnostic test on Masters student James Bell
Measuring the health of nerves in people is tricky business. Current electrical diagnostic tests are a step in the right direction; however, a barrier in this field of research is the time and high level of expertise needed to interpret the copious amounts of data these tests create. With the majority of his research looking at the impact exercise has on degenerative nerve disease, Faculty of Physical Education and Recreation associate professor Kelvin Jones knows that better electrical diagnostics are needed to measure nerve health.
Thanks to a recent Natural Sciences and Engineering Research Council (NSERC) Discovery Grant, Jones aims to use machine learning-better known as Artificial Intelligence (AI)-to help create a definitive nerve test. An AI program, which will be created by Jones' Masters student James Bell, will be able to analyze this difficult information and create a tool to diagnose diseases that create nerve damage in the body, and measure the benefits from interventions like exercise.
"We've seen solid evidence that shows the results of electrical diagnostics would be very useful for individuals with nerve damage," says Jones "By feeding the information into a computer program, James will create an algorithm for both healthy and unhealthy nerves, essentially teaching the system to differentiate between the two."
Over the next two years, James will input data from healthy control patients collected at UAlberta-and in labs in Australia, Japan, Ireland and England-into the AI program, compiling the first-ever international normative nerve database. This database will act as a baseline in which all data will be tested against. Because the AI program will be given algorithms for both healthy and unhealthy nerves, it will be able to tell almost immediately if the data from the electrical diagnostics is typical or atypical.
Once the normative nerve database is complete, Jones will then implement a computer model of nerve function that mimics the electrical behavior of the nerves in the body. By creating hundreds of thousands of nerve-function scenarios, Jones can then run an individual's nerve test results against these computer models to best determine what sort of nerve damage or disease this person may have.
Jones explains that for individuals with degenerative nerve diseases like ALS, having electric diagnostic data interpreted immediately should speed diagnosis by providing an additional biomarker for the disease. "Testing for ALS involves a number clinical tests, neuroimaging, blood and fluid samples to rule out other diseases as opposed to a single specific test for the disease. This process takes 12-18 months before coming to a diagnosis. A year to a year-and-a-half is crucial time for individuals with ALS and their families."
Jones hopes that the data will also help determine a prognosis and provide personalized health options. Knowing how aggressive the progression of diseases like ALS is can go a long way in determining a treatment plan. According to Jones' previous research, evidence showed exercise or sedentary behavior affects the rate of ALS progression. When the nerve damage is too far gone, or was progressing too quickly, results showed that exercise did not made a significant impact. By understanding how the disease is progressing, those living with ALS can make an informed decision on how they would like to spend their time.
While research around exercise as it relates to muscle health, heart health, body composition, bone density and cognitive function has been happening for decades, investigating its effects on nerve health is fairly new area of study.
"There is now a focus on looking at both the structure and physiology of nerves to see the effects of exercise. But in order to do that, we definitely need to know what a healthy nerve really looks like. Building a normative database and creating an AI program to quickly interpret data can go along way in moving this area of research forward."