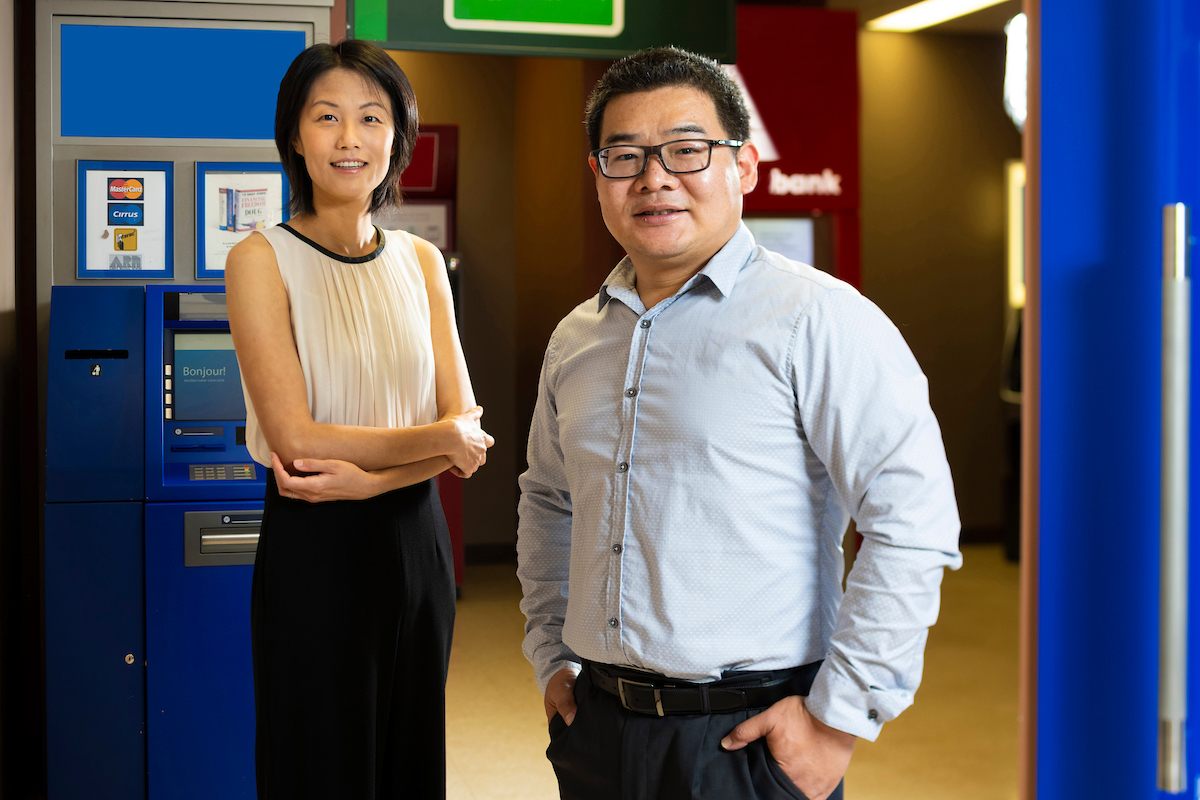
Statisticians and faculty members Bei Jiang (left) and Linglong Kong (right). Photo credit: John Ulan
Millions of Canadians trust these financial institutions not only with their savings, but with their data. As the financial industry looks to make ever better data-driven decisions, tools based on artificial intelligence (AI) and machine learning are emerging as the focus—and the University of Alberta’s unique strengths in the fields of AI and statistical analysis are putting campus expertise at the forefront of the next big movement in money.
“The financial industry wants to improve their predictions using new methods,” says Bei Jiang, (’08 MSc) (mathematical and statistical sciences). “Machine learning is one of the AI tools especially well-suited to their wealth of data.“
Machine learning refers to algorithms for analyzing large amounts of data, revealing unapparent connections. Jiang’s research applies this technology to debt collection optimization, open banking initiatives, and data privacy. Her team’s research partnerships have included one with a regional bank in the province. The team is helping to apply statistical analysis so that the bank can better understand a small business’s financial health when deciding whether to approve a loan.
“This is a zero-one decision: approving or not. But it’s a decision that requires robust data analysis, a major focus of my research,” explains Jiang. “The financial industry’s data is very complex—they can’t just look at the age or gender of a customer to determine their financial health.” They have to look at “a variety of points of data that are all important predictors of whether a particular loan is a good investment.”
Data and Goliath
Analyzing that complex data is the main challenge facing the industry, and one that’s a perfect fit for both machine learning algorithms and statisticians.
“We look at data with complex structures and develop tools to conduct estimation and prediction and to make other inferences based on that data,” says Linglong Kong (’09 PhD) (mathematical and statistical sciences). “That is a focus of AI in the form of statistical machine learning.”
Kong’s research is helping industry partners understand the financial well-being of their customers, and his team has collaborated with an Edmonton-based financial institution on a project to identify the most effective ways to retain and attract new customers. “Many industries are now looking at how to make use of machine learning and to apply algorithms to their data,” says Kong. “But the majority of the work should happen before even entering data into an algorithm, asking questions like ‘what is important to this application?’ It’s not just doing it—it’s doing it right.”
Held in trust
AI is a strength of the University of Alberta’s Faculty of Science—a field in which our researchers are among the best in the world. Since 2000, metrics-based CSRankings.org has placed campus AI publications in the top three in the world—and at the top in North America. But Kong explains that research partnerships with the financial industry aren’t just based on campus expertise.
“When we create these algorithms for industry, we’re creating them from the ground up and building them right, by developing techniques that adhere to three pillars: they are explainable, sustainable, and responsible. These are factors that are incredibly important to the financial industry.”
Explainability
Imagine you operate a small business. Eager to expand your operations, you apply for a loan at your bank. If you hear back that you’ve been denied, the first question on your mind is likely “why?”
“When a financial institution makes a decision, it isn’t sufficient to simply tell a customer ‘the algorithm said so,’” says Kong. “There’s a need—a legal need—to be able to explain that decision to the customer.” This is one of the pillars of Kong’s research and one of the primary advantages he sees for financial institutions in working with statisticians. When designing tools for the financial industry, the objective is not only creating algorithms capable of making recommendations but creating ones that highlight what led to that recommendation, helping to demystify artificial intelligence.
“It’s important to be able to look past the result and explain to the customer why a decision has been made, not only for the customer experience but also for that decision to stand up in a court of law,” explains Kong.
Sustainability
Building custom tools for the financial industry is not a quick process. So when designing a tool for the financial industry, says Kong, it’s best to build a Swiss Army knife. “You want an algorithm that is able to adapt when a situation changes. If a bank gets new data about their customers and the algorithm needs to be built from scratch and retrained every time, that’s unsustainable design.”
It might seem like an obvious concept: after all, we're used to the idea of recycling. But designing a robust tool from the beginning is another way that UAlberta AI experts are providing long-term value to the financial industry.
Responsibility
The last pillar extends beyond the financial industry: the responsible implementation of AI tools in all facets of their application. Examples of hastily implemented machine learning algorithms trained on biased data abound—highlighting the importance of both data analysis before a project starts, and the applications of these tools. Campus research in this area includes Project BIAS, an international project using the power of AI to combat ethnic and gender bias in the labour market in areas such as job advertising, hiring, and networking.
The future of finance
As the financial industry prioritizes finding the best, most effective ways to make data-driven decisions, the University of Alberta’s expertise in artificial intelligence makes it a powerful partner in these valuable industry partnerships.
“Artificial intelligence and machine learning are powerful tools for our business moving forward,” says Mark Wagner, vice-president (analytics practice & corporate functions) at Scotiabank. “Our partnerships with academia provide a unique pipeline for idea generation and innovation, fostering the next generation of talent and building communities of practice for artificial intelligence and machine learning.”
World-class expertise
The Faculty of Science includes experts in AI, computer vision, data analytics, machine learning, mathematical finance, natural language processing, and robust statistics. Meet some of the other minds behind UAlberta AI research and the partnerships strengthening Alberta’s expertise in this important field:
- Chris Frei (mathematical and statistical sciences) studies quantitative finance, risk management, and mathematical economics. He is also an expert in digital and cryptocurrencies.
- Eleni Stroulia (computing science) examines how collaborative, model-driven software can improve information and communication technology.
- Michael Bowling (computing science) works with industry to develop robust decision-making tools using customer data.
- Omid Ardakanian (computing science) is building a data analytics platform to support the work of collaborators in finance.
The Alberta advantage: key projects and partnerships
- AltaML
- Alberta-based machine learning company AltaML is focused on building applications powered by applied machine learning to drive innovation. The company, founded by UAlberta alumni, has funded a professorship in natural language processing in the Department of Computing Science, and also provides support for programming at the Faculty of Science Student Innovation Centre.
- Scotiabank
- The Scotiabank Artificial Intelligence Research Initiative, housed in the Faculty of Science, is designed to enhance AI research to understand and build practical tools and predictive models for fraud detection and speech-to-text in analytics. Scotiabank is also supporting the growth of diversity and women in science, technology, engineering, and mathematics (STEM) at UAlberta.
- Servus Credit Union
- Servus Credit Union is collaborating with UAlberta scientists on research projects in data science, AI, machine learning, natural language processing, and related areas. The partnership includes joint research projects and opportunities to apply graduate student research to real-world financial challenges.