A University of Alberta research team has successfully used machine learning as a tool for earlier detection of attention deficit hyperactivity disorder (ADHD) in kindergarten students.
In a recent study published in the journal PLOS Digital Health, the team analyzed de-identified provincial health records and teachers’ developmental assessments to accurately predict which students would go on to be diagnosed with ADHD within the following four years.
“By applying machine learning to this data, we can predict ADHD several years down the road for these children,” explains principal investigator Bo Cao, associate professor of psychiatry, adjunct professor of computing science and Canada Research Chair in Computational Psychiatry.
“The long-term goal of the research is to focus on high-risk populations and see if there are modifiable factors so that we can reduce the risk,” Cao says.
“Unidentified ADHD can have a huge impact on the developmental trajectory of kids because if they can’t follow the class in kindergarten, then it just gets harder in the later grades,” says first author Yang Liu, research associate in the Computational Psychiatry Lab whose work is supported by the 2023 Brain and Behaviour Research Foundation Young Investigator Grant.
The research team also included contributors from Alberta Health and the Alberta Machine Intelligence Institute.
ADHD is the most common neurodevelopmental disorder in children, affecting about five to nine per cent of children and three to five per cent of adults, according to the Centre for ADHD Awareness, Canada.
There are three types of ADHD: hyperactive, inattentive and a combination of the two. Symptoms can include impulsivity, learning difficulties and poor emotional self-regulation. The average age at diagnosis is seven for boys and 12 for girls. In Alberta, treatments offered include behaviour therapy, counselling and medication.
The team analyzed records for 23,247 children who were in kindergarten in Alberta in 2016. Children who had already been diagnosed with ADHD were not included. Within the following four years, 1,680 or 7.2 per cent of the children studied received a diagnosis of ADHD.
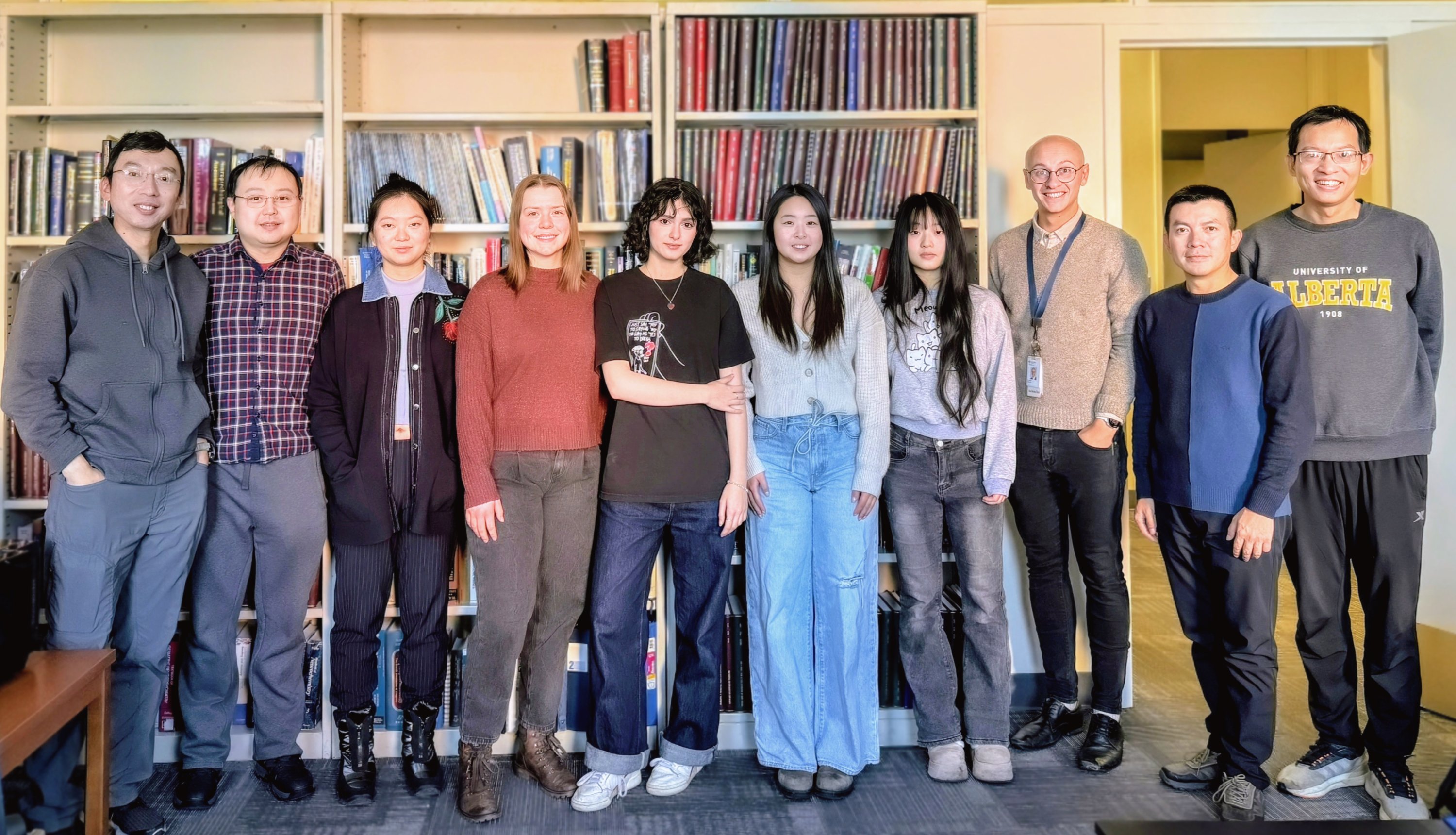
The study team cross-linked administrative health data with results from a developmental assessment tool used by teachers, the Early Development Instrument. The questionnaire is completed by kindergarten teachers to measure whether children are meeting developmental expectations for physical health, social competence, emotional maturity, language and cognitive development, communication skills and general knowledge. The results are grouped to give a snapshot of how children are doing across schools, neighbourhoods, cities or provinces.
The health data included records such as health issues at birth, past mental health of the mothers and number of emergency department visits by the children, as well as demographic information by neighbourhood such as household size, average percentage of income spent on housing, and education levels.
The algorithm predicted future ADHD diagnoses with an Area Under the Curve (AUC) of 0.81 when it used the combination of data from both the health records and the assessment tool. The AUC is a metric used to evaluate model prediction performance, with an AUC of 1 representing perfect performance.
The team reports the top predictive factors for a lower risk of future ADHD diagnosis were teacher-reported good learning strategies and social skills, speaking English or French as a second language (ESL/FSL), and being female. The predictive factors for a higher risk included inattentive classroom behaviour, a history of mental health hospital visits, higher neighbourhood education, maternal mental health issues, higher relative housing spend and larger household size.
Cao and Liu caution that none of these factors can be considered in isolation, as they are likely interdependent and their impact warrants future study. For example, teachers and parents may be more alert to ADHD in boys compared with ESL students or girls, leading to a relative delay in diagnosis for those groups. The team hopes to develop more specialized predictive models for these subgroups of students and also follow them for a longer time.
Cao has published similar papers using machine learning and health data to predict opioid use disorder, depression onset and other mental health issues. He notes that while it is encouraging to see that AI can provide diagnostic insights, for now many of its potential applications are still at the research stage.
“We know that using data and machine learning can add value, but exactly what is that value? What are the risks and how can this model be used in the real world?” he asks. “We need to engage stakeholders, community members and people with lived experiences to be sure we respect privacy and different cultures, avoid stigma, all kinds of things.
“I hope this model will serve as a stepping stone for earlier interventions, enabling children with ADHD to receive support when it matters most,” says Cao.
Cao is co-director of the Computational Psychiatry Lab at the U of A, a cross-disciplinary team that includes researchers from the Faculty of Medicine & Dentistry and the Faculty of Science. He is a member of AI4Society, the Neuroscience and Mental Health Institute, the SMART Network and the Women and Children’s Health Research Institute.